Adaptive Test Selection for Deep Neural Networks
May 27, 2022·
,,,,,·
0 min read

Xinyu Gao
Yang Feng
Yining Yin
Zixi Liu
Zhenyu Chen
Baowen Xu
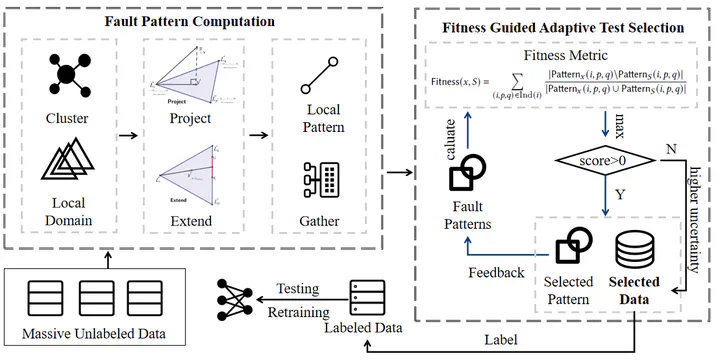
Abstract
Deep neural networks (DNNs) have achieved tremendous development in the past decade. While many DNN-driven software applications have been deployed to solve various tasks, they could also produce incorrect behaviors and result in massive losses. To reveal the incorrect behaviors and improve the quality of DNN-driven applications, developers often need rich labeled data for the testing and optimization of DNN models. However, in practice, collecting diverse data from application scenarios and labeling them properly is often a highly expensive and time-consuming task.
In this paper, we propose an adaptive test selection method, namely ATS, for deep neural networks to alleviate this problem. ATS leverages the difference between the model outputs to measure the behavior diversity of DNN test data. It aims at selecting a subset with diverse tests from a massive unlabeled dataset. We experiment with ATS using four well-designed DNN models and four widely-used datasets in comparison with various kinds of neuron coverage. The results demonstrate that ATS can significantly outperform all test selection methods in assessing both fault detection and model improvement capability of test suites. It is promising to save the data labeling and model retraining costs for deep neural networks.
In this paper, we propose an adaptive test selection method, namely ATS, for deep neural networks to alleviate this problem. ATS leverages the difference between the model outputs to measure the behavior diversity of DNN test data. It aims at selecting a subset with diverse tests from a massive unlabeled dataset. We experiment with ATS using four well-designed DNN models and four widely-used datasets in comparison with various kinds of neuron coverage. The results demonstrate that ATS can significantly outperform all test selection methods in assessing both fault detection and model improvement capability of test suites. It is promising to save the data labeling and model retraining costs for deep neural networks.
Publication
44th IEEE/ACM International Conference on Software Engineering
(ICSE 2022, CCF-A)
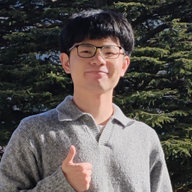