Benchmarking Robustness of AI-Enabled Multi-sensor Fusion Systems: Challenges and Opportunities
Dec 9, 2023·
,,,,,·
0 min read

Xinyu Gao
Zhijie Wang
Yang Feng
Lei Ma
Zhenyu Chen
Baowen Xu
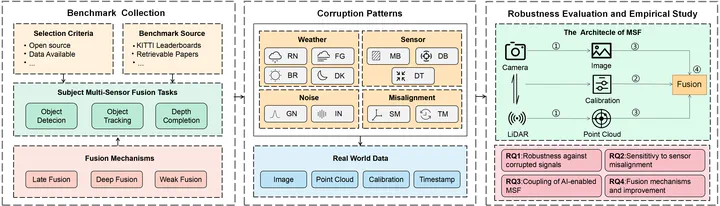
Abstract
Multi-Sensor Fusion (MSF) based perception systems have been the foundation in supporting many industrial applications and domains, such as self-driving cars, robotic arms, and unmanned aerial vehicles. Over the past few years, the rapid progress in data-driven artificial intelligence (AI) has led to an increasing trend of enhancing MSF systems with deep learning techniques to further improve performance, particularly in intelligent systems and their perception components. Although numerous AI-enabled MSF perception systems and techniques have been proposed, limited benchmarks focusing on MSF perception are publicly available. Given that many intelligent systems, such as self-driving cars, operate in safety-critical contexts where perception systems play a crucial role, there is an urgent need for a deeper understanding of the performance and reliability of these MSF systems.
To bridge this gap, we take an initial step in this direction and construct a public benchmark of AI-enabled MSF-based perception systems, covering three commonly adopted tasks: object detection, object tracking, and depth completion. Based on this benchmark, we design 14 common and realistic corruption patterns to synthesize large-scale corrupted datasets, enabling a comprehensive evaluation of MSF systems’ robustness and reliability. Through our large-scale evaluation, we identify the following key findings: (1) existing AI-enabled MSF systems are not robust enough against corrupted sensor signals; (2) small synchronization and calibration errors can cause AI-enabled MSF systems to fail; (3) AI-enabled MSF systems are typically tightly coupled, meaning that errors from an individual sensor can lead to a system-wide failure; (4) the robustness of MSF systems can be improved by enhancing fusion mechanisms. Our results highlight the vulnerabilities of current AI-enabled MSF perception systems, urging researchers and practitioners to prioritize robustness and reliability in their designs. Our benchmark, code, and detailed evaluation results are publicly available at https://sites.google.com/view/ai-msf-benchmark.
To bridge this gap, we take an initial step in this direction and construct a public benchmark of AI-enabled MSF-based perception systems, covering three commonly adopted tasks: object detection, object tracking, and depth completion. Based on this benchmark, we design 14 common and realistic corruption patterns to synthesize large-scale corrupted datasets, enabling a comprehensive evaluation of MSF systems’ robustness and reliability. Through our large-scale evaluation, we identify the following key findings: (1) existing AI-enabled MSF systems are not robust enough against corrupted sensor signals; (2) small synchronization and calibration errors can cause AI-enabled MSF systems to fail; (3) AI-enabled MSF systems are typically tightly coupled, meaning that errors from an individual sensor can lead to a system-wide failure; (4) the robustness of MSF systems can be improved by enhancing fusion mechanisms. Our results highlight the vulnerabilities of current AI-enabled MSF perception systems, urging researchers and practitioners to prioritize robustness and reliability in their designs. Our benchmark, code, and detailed evaluation results are publicly available at https://sites.google.com/view/ai-msf-benchmark.
Publication
Proceedings of the 31st ACM Joint European Software Engineering Conference and Symposium on the Foundations of Software Engineering
(ESEC/FSE 2023, CCF-A)
Award
ACM SIGSOFT Distinguished Paper Award